When we first began writing about the role technology could play in education, we focused on the idea that technology provided tools for thinking and learning. This idea came from the work of David Jonnasen and his book MindTools. We built on his observation that technology tools many folks used to accomplish tasks in their everyday lives (writing to communicate, spreadsheets to organize and ask questions of numerical data, etc.) could be used in classrooms not just with the goal of learning how to use the tools, but to develop an understanding of the concepts and skills important to content areas by applying the technology tools to the processing of content area information. Our first textbook used the umbrella of technology integration to describe this general approach, expanded the list of technology tools that could be useful, and created a theoretical and research-based framework based on authentic tasks, constructivism, and contextualized learning to explain and justify such tasks.
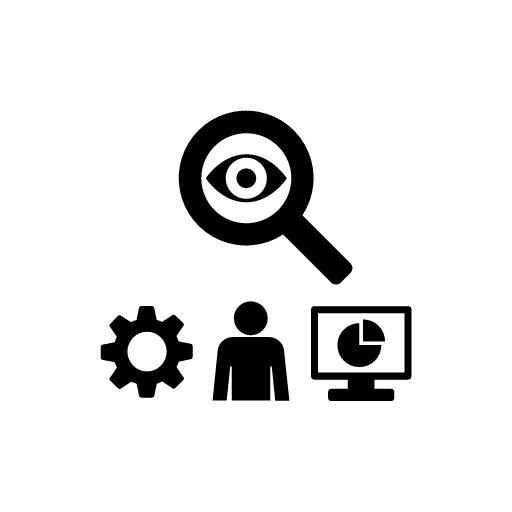
I don’t know if blog posts are the place for exploring what I consider core concepts that drive curriculum development and classroom practice, but I want to try out some big ideas that I think have value and are not being considered by enough educators.
Let me start with a comparison. Coding/programming has received a great deal of recent attention as identifying concrete tasks that can be used in classrooms with proposed benefits that are either not presently achieved or that can generalize in ways that make a focus on such tasks more efficient than existing classroom activities. Based on these arguments educators and administrators have been lobbied to turn over classroom time to coding/programming experiences. The benefits of this reallocation could result in a) the development of an important vocational skill (coding), b) deeper insight into how technology applications actually work (technology literacy), and c) the development of computational thinking. As you move through this list, I would argue that the proven benefits become more difficult to demonstrate. There is a difference between speculating about benefits and demonstrating these benefits exist.
OK, so coding for all has caught people’s attention and this attention has resulted in real change in how students spend time. There are secondary issues that must follow if educators continue to implement classroom experiences based on a focus on this category of classroom activities. For example, at some point, it makes sense that educators providing such experiences actually be prepared to do so and their competency in this area be evaluated as a component of their preparation to teach. We are yet to reach the point at which this is happening at a meaningful level.
The issue I am trying to address concerns how a certain type of classroom experience gains leverage in determining how classroom time is spent. Is it based on the identification of skills that are to result from education and a consideration of what learning experience best develop these skills? I don’t have the sense that computational thinking was identified as something all students need and an effort was then followed to determine how best to develop such skills. The emergence of computational thinking seemed more a justification that followed something already gaining momentum to add more pressure to the cause.
I understand the public’s interest in technology and the mystery in many people’s minds as to how the magic of technology is accomplished. OK – this fascination in some areas could result in a preparatory course at the secondary level and the opportunity to major in a discipline in college. How did we get from such possibilities to the argument that all students need to develop computational thinking? As might now be obvious, I don’t think computational thinking is an actual thing and the concept is just an area-specific version of problem-solving. Why stop with coding? One could easily argue that compositional thinking as exhibited by those heavily involved as writers and promoted by educators as writing across the curriculum should be recognized for its proven general benefits, but is commonly ignored because committing more time to writing activities is less trendy. The generalizability of higher-order cognitive skills has long been controversial. We call this transfer and achieving transfer from a specific application of a cognitive skill to a more general application of this skill is tricky and difficult to demonstrate. It simply does not happen automatically with the amount of experience schools can devote to coding and the existing practices of teaching coding are not designed to transfer whatever computational thinking is expected to emphasize.
All this aside, I think I can make a better argument for a completely different area of skills, knowledge, and dispositions I think have greater generality. Part of the challenge I see in my effort is the generation of a cutesy name. I can’t come up with something for what I have in mind that is the equivalent of computational thinking. Perhaps I will eventually make an effort, but I will first offer the background for this proposal.
What I have in mind can be identified at the level of application in such claims as data-driven or empirically supported. As consumers, clients, patients, etc., we want to believe that the value, accuracy, etc. of the experiences, information, etc. we are provided have been established. How such goals are met depends on the discipline, but all disciplines have processes based in the collection of data through processes I like to describe as methods. We are probably most familiar with the approach we associate with “the sciences” and that abstract model we call the scientific method. Still, historians have data and apply processes and dispositions to these data to generate accounts of history that they argue are accurate and unbiased. Economists and political scientists do the same. Marketers attempt to identify public opinions in order to make decisions on product preferences and persuasive approaches. Data collection, analysis, and practices based on the consequences of collection and analysis are everywhere.
At best, we give lip service to the scientific method in K-12 science instruction. Students typically follow carefully scripted labs and have no experience designing experiments (methods) to test ideas (hypotheses) through the collection and analysis of data (statistics).
Like computational thinking, I see the benefits on multiple levels. Methods and analytical frameworks vary with domain. There is more. With experience, you learn not just the methods of a given domain, but also the limits of these methods. An example familiar to many is the use of a conclusion generated from an experiment that does not involve manipulation in arguing a causal relationship. This problem is commonly described as “correlation is not equal to causation”. Another example is that I would describe as anecdotal reasoning. For example, a politician tells the story of an individual in justifying some policy. The example may be accurate but the conclusion that this example is the most common result of similar circumstances is unproven. The skills involved in the specification of a problem and the establishment of a methodology to collect data relevant to this problem and then the evaluation of what such data indicate are everywhere. Where and when do we acquire such skills and in how many areas are such skills developed? We should develop such skills even when we do not apply them directly as we need to evaluate the cases based in data that others offer us to influence our behavior.
This post is getting very lengthy and I am guessing readers want me to get past my rationale to something that is actionable. I have some very concrete suggestions for classrooms in mind. I propose that educators take a look at the content they teach and think about the skills they are trying to develop and begin to ask the following questions:
- What data are relevant to the topics I teach? What data can my students collect that have some bearing on these topics?
- After relevant examples of data have been identified, the thinking then should move to the methods that can be used to collect such data and what about these methods should be considered that could limit accurate conclusions being reached.
- Finally, educators should consider the dispositions that must accompany data collection and analysis. What attitudes encourage critical thinking and openness to the examination of personal beliefs that data collection might challenge?
Once you understand these goals, data sources, data collection and analysis methods, and desirable dispositions are everywhere. You collect data when you go to the library or search online to answer a question. What search tactics increase the likelihood useful information will emerge from your search? What commitments to thinking about this information will allow you to discount misinformation and use valid information to challenge existing beliefs? You collect data when you create a questionnaire to identify the choice a class prefers among multiple options. How can the questions in the questionnaire be written to avoid bias? The examples of opportunities are limitless, authentic, contextualized, and focused on skills necessary throughout life. This is a mindset that is consistent with existing curriculum goals, but emphasizes skills and methods of developing these skills that are seldom emphasized.
With this position statement as a starting point, I intend over the next several weeks to identify some examples of methodology, data collection, and analysis that encourage others to imagine their own possibilities.
More related content to follow.
One thought on “Educational goals shape classroom practice”