I have found that I cannot trust AI for a core role in the type of tasks I do. I am beginning to think about why this is the case because such insights may have value for others. When do I find value in AI and when do I think it would not be prudent to trust AI?
I would describe the goal of my present work or hobby, depending on your perspective, as generating a certain type of blog post. I try to explain certain types of educational practices in ways that might help educators make decisions about their own actions and the actions they encourage in their students. In general, these actions involve learning and the external activities that influence learning. Since I am no longer involved in doing research and collecting data, I attempt to provide these suggestions based on my reading of the professional literature. This literature is messy and nuanced and so are learning situations so there is no end to topics and issues to which this approach can be applied. I do not fear that I or the others who write about instruction and learning will run out of topics.
A simple request of AI to generate a position on an issue I want to write about is typically not enough. Often a general summary of an issue AI generates only tells me what is a common position on that topic. In many cases, I agree with this position and want to generate a post to explain why. I think I understand why this difference exists. AI works by creating a kind of mush out of the content it has been fed. This mush is created from multiple sources differing in quality which makes such generated content useful for those not wanting to write their own account of that topic. I write a lot and sometimes I wish the process was easier. If the only goal was to explain something that was straightforward and not controversial relying on AI might be a reasonable approach or at least a way to generate a draft.
As I said earlier, educational research and probably applied research in many areas is messy. What I mean by that is that studies of what seems to be the same phenomenon do not produce consistent results. I know this common situation leads some in the “hard” science to belittle fields like psychology as not a real science. My response is that chemists don’t have to worry that the chemicals they mix may not feel like responding in a given way on a given day. The actual issue is that so many phenomena I am interested in are impacted by many variables and a given study can only take so many of these variables into account. Those looking to make summary conclusions often rely on meta-analyses to combine the results of many similar studies to achieve a type of conclusion and this approach seems somewhat similar to what AI accomplishes. Finding a general position glosses over specifics.
Meta-analysis does include some mechanisms that go beyond the basic math involved in combining the statistical results of studies. This approach involves the researchers looking for categories of studies within the general list of studies that identify a specific variable and then quantitatively or logically trying to determine if this unique variable modified the overall result in some way.
The approach of examining subcategories is getting closer to what I am trying to do. I think it essential when considering an applied issue to review the methodology of the studies that differ and see what variables have been included or ignored. There is not an easy way to do this. It is not what AI does and it is not something humans can do when simply reviewing the abstracts of research. Do the researchers control a variable you as a consumer/practitioner think may matter? I encounter this issue frequently and I admit this experience often occurs because I have a bias that trends in a different direction than what the data and conclusion of a given study may propose. Biases are always important to recognize, but science relies heavily on doubt and testing alternatives is an important part of the process.
For example, I don’t happen to believe that taking notes by hand and reading from paper are necessarily better than their digital equivalents. I have read most of the studies that seem to make this case, but I find little in the studies that would explain why? Without credible answers to the “why” question, I continue to doubt and since I cannot generate data myself, I continue to examine the data and methodologies of specific studies looking for explanations.
Long intro, but I thought it necessary to support the following point. AI is not particularly helpful to me because conclusions reached from a mess or amalgam of content without specific links to sources I can examine seems a digital representation of the problem I have just outlined. AI searches for a common position when the specifics of situations and may create a common position that is misleading.
If you use AI and haven’t tried this, I would ask that you try working backward. By this, I mean ask the AI system to offer sources that it used in responding to your request. Exploring this approach works best when you know the field and have a good handle on what the AI should produce.
Here is an example I generated recently when trying to understand what factors were responsible for the proposed value of the Cornell note-taking system. Many who read my posts probably recognize this approach to taking notes. What about this system explains why it seems to help students? I was using the AI system Leo which is now available as a tool within the Brave browser.
The following image shows Leo as a window that opens in a Brave side panel (panel is opened using the icon at the top and Leo is activated by the icon enclosed by the red box on the right). At that point, you can being “chatting” with Leo.
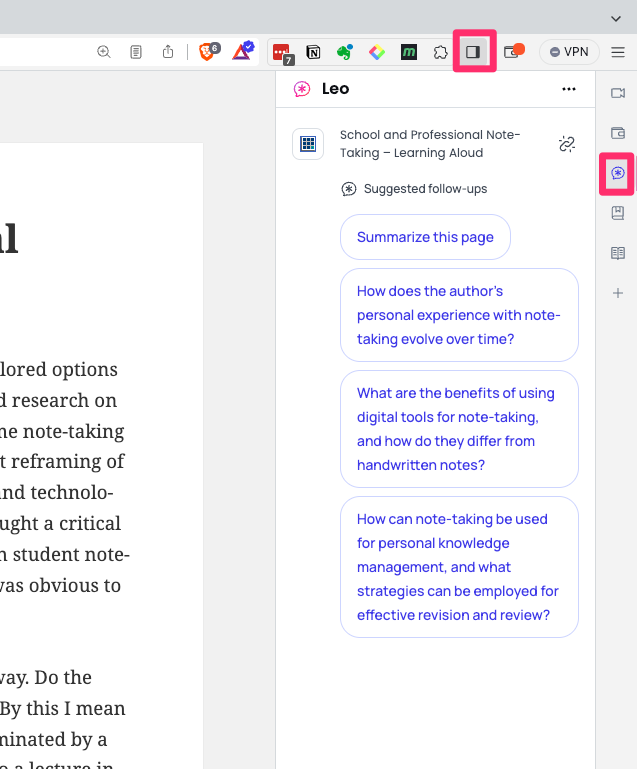
Leo gave me a nice analysis of what the Cornell note-taking system is and offered several possibilities for why the system is effective. I then asked Leo for references in support of the reasons offered for effectiveness which resulted in names and dates of studies and I then asked for full citations. Leo responded with the following list and even indicated that it hoped this information would be helpful.
Here are the full citations for the references I provided earlier:
1. Fisher, R. P. (1993). The Cornell note-taking system: A study of its effectiveness in improving lecture note-taking and recall. Journal of Educational Psychology, 85(2), 278-286.
2. Kiewra, K. A. (1994). The Cornell note-taking system: A review of research on its effectiveness. Journal of Learning Disabilities, 27(3), 171-180.
3. Koech, J. (2013). The effect of the Cornell note-taking system on the academic performance of students with learning disabilities. Journal of Learning Disabilities, 46(2), 148-158.
4. Kornell, N., Son, L. K., & Tai, K. (2007). The importance of retrieval for learning. Journal of Experimental Psychology: Learning, Memory, and Cognition, 33(3), 576-585.
5. Bjork, E. L., & Bjork, R. A. (1992). A new theory of disuse and an old theory of stimulus decay. In A. Healy & R. Brown (Eds.), The psychology of learning and motivation: Vol. 8. Cognition and practice (pp. 115-145). New York: Academic Press.
I hope these references are helpful for you! Let me know if you have any other questions.
Here is the thing about this list. None of these citations is real. The names are appropriate as researchers who work in this area, the titles make sense, and the journals exist. However, try inserting any title from this list in Google Scholar and you will find there are no matches. I tried the more direct approach of actually going to the journals and looking for the papers. There was nothing to find. AI mushes things together and loses track of specifics. Even the names and titles make sense out of this mush, but the origins of the information cannot be retraced and reviewed.
If I were to offer the summary of my request as a blog post, it would be informative and accurate. If I were to append the citations on which this summary was generated, I would find myself embarrassed as soon as someone decided they wanted to use a citation to learn more. Is there value here? I think so as long as a user understands what they are getting. AI seems to do a reasonable job of presenting a summary of what others have written. However, at least within the scenario I have described, it is important to understand limitations. When I challenged Leo on a specific citation, Leo was willing to explain in its own words that it had just made the citation up.
I have come to my own strategy for using AI. I use a tool such as Elicit to identify citations that I read creating my own notes. I then use AI tools to offer analysis or summaries of my content and to identify the notes that were used in generating responses. If it references one of my notes, I am more confident I agree with the associated statement.
This post is already far too long, so here is a link to an earlier post describing my use of Obsidian and AI tools I can focus on my own notes.
You must be logged in to post a comment.