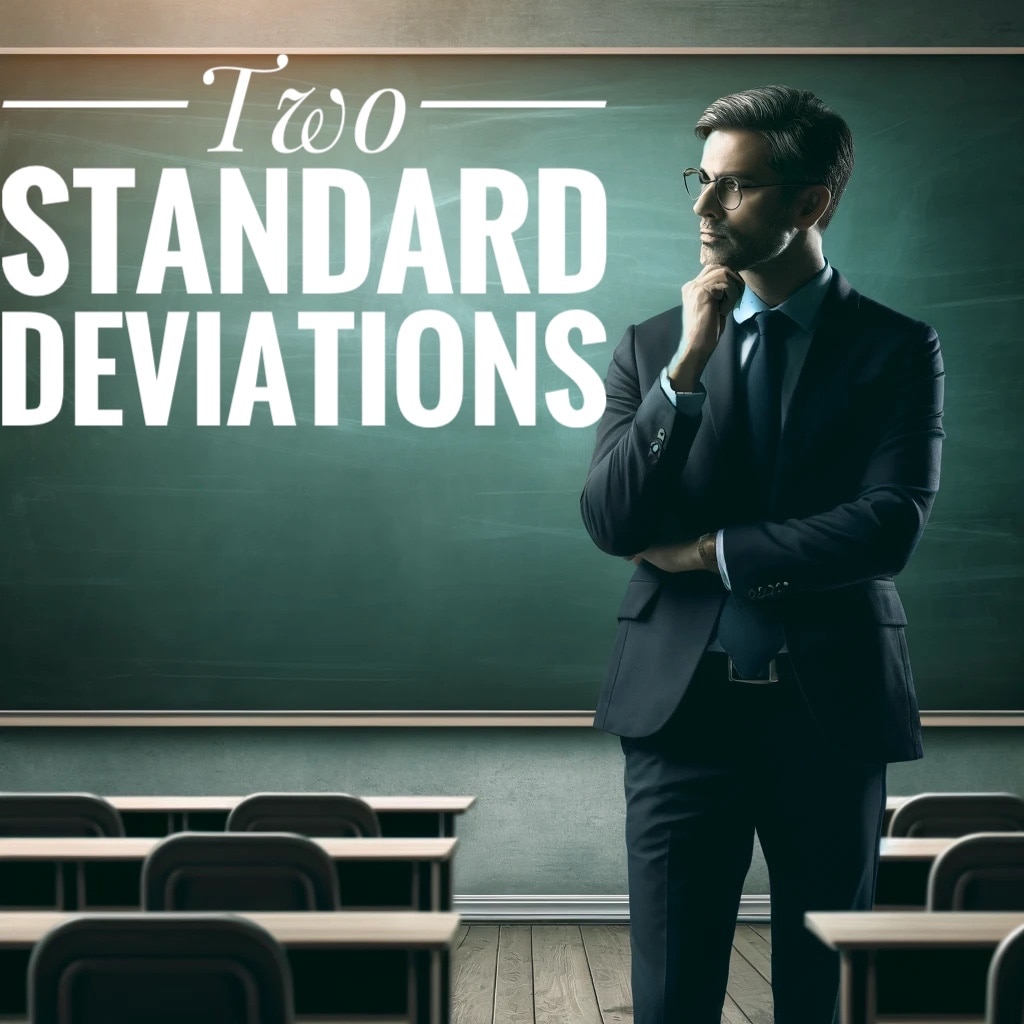
This is an erratum in case my previous posts have misled anyone. I looked up the word erratum just to make certain I was using the word in the correct way. I have written several posts about AI tutoring and in these posts, I made reference to the effectiveness of human tutoring. I tend to provide citations when research articles are the basis for what I say and I know I have cited several sources for comments I made about the potential of AI tutors. I have not claimed that AI tutoring is the equal of human tutoring, but suggested that it was better than no tutoring at all, and in so doing I have claimed that human tutoring was of great value, but just too expensive for wide application. My concern is that I have proposed that the effectiveness of human tutoring was greater than it has been actually shown to be.
The reason I am bothering to write this post is that I have recently read several posts proposing that the public (i.e., pretty much anyone who does not follow the ongoing research on tutoring) has an inflated understanding of the impact of human tutoring (Education Next, Hippel). These authors propose that too many remember Bloom’s premise of a two-sigma challenge and fail to adjust Bloom’s proposal that tutoring has this high level of impact on student learning to what the empirical studies actually demonstrate. Of greater concern according to these writers is that nonreseachers including educational practitioners, but also those donating heavily to new efforts in education continue to proclaim tutoring has this potential. Included in this collection of wealthy investors and influencers would be folks like Sal Kahn and Bill Gates. I assume they might also include me in this group while I obviously have little impact in compared to those with big names. To be clear, the interest of Kahn, Gates, and me is really in AI rather than human tutoring, but we have made reference to Bloom’s optimistic comments. We have not claimed that AI tutoring was as good as human tutors, but by referencing Bloom’s claims we may have led to false expectations.
When I encountered these concerns, I turned to my own notes from the research studies I had read to determine if I was aware that Bloom’s claims were likely overly optimistic. It turns out that I had read clear indications identifying what the recent posters were concerned about. For example, I highlighted the following in a review by Kulik and Fletcher (2016).
“Bloom’s two sigma claim is that adding undergraduate tutors to a mastery program can raise test scores an additional 0.8 standard deviations, yielding a total improvement of 2.0 standard deviations.”
My exposure to Bloom’s comments on tutoring originally had nothing to do with technology or AI tutoring. I was interested in mastery learning as a way to adjust for differences in the rate of student learning. The connection with tutoring at the time Bloom offered his two-sigma challenge was that mastery methods offered a way to approach the benefits of the one-to-one attention and personalization provided by a human tutor. Some of my comments on mastery instruction and the potential of technology for making such tactics practical are among my earlier posts to this site. Part of Bloom’s claim being misapplied is based on his combination of personalized instruction via mastery tactics with tutoring. He was also focused on college-aged students in the data he cited. My perspective reading the original paper many years ago was not “see how great tutoring is”. It was more tutoring on top of classroom instruction is about is good as it is going to get and mastery learning offers a practical tactic that is a reasonable alternative.
As a rejoinder to update what I may have claimed, here are some additional findings from the Kulik and Fletcher meta-analysis (intelligent software tutoring).
The studies reviewed by these authors show lower benefits for tutoring when outcomes are measured on standardized rather than local tests, sample size is large, participants are at lower grade levels, the subject taught is math, a multiple-choice test is used to measure outcomes, and Cognitive Tutor is the ITS used in the evaluation.
However, on a more optimistic note, the meta-analysis conducted by these scholars found that in 50 evaluations intelligent tutoring systems led to an improvement in test scores of 0.66 standard deviations over conventional levels.
The two sources urging a less optimistic perspective point to a National Board of Educators Research study (Nickow and Colleagues, 2020) indicating that human tutoring for K-12 learners was approximately .35 sigma. This is valuable, but not close to the 2.0 level.
Summary
I have offered this update to clarify what might be interpreted based on my previous posts, but also to provide some other citations for those who now feel the need to read more original literature. I have no idea whether Kahn, Gates, etc. have read the research that would likely indicate their interest in AI tutoring and mastery learning was overly ambitious. Just to be clear I had originally interpreted the interest of what the tech-types were promoting as mastery learning (personalization) which was later morphed into a combination with AI tutoring. This combination was what Bloom was actually evaluating. The impact of a two-sigma claim when translated into what such an improvement would actually mean in terms of rate of learning or change in a metric such as assigned grade seems improbable. Two standard deviations would move an average student (50 percentile) to the 98th percentile. This only happens in Garrison Keeler’s Lake Wobegon.
References:
Bloom, B. S. (1984). The 2 sigma problem: The search for methods of group instruction as effective as one-to-one tutoring. Educational researcher, 13(6), 4-16.
Kulik, J. A., & Fletcher, J. D. (2016). Effectiveness of intelligent tutoring systems: a meta-analytic review. Review of educational research, 86(1), 42-78.
Nickow, A., Oreopoulos, P., & Quan, V. (2020). The impressive effects of tutoring on prek-12 learning: A systematic review and meta-analysis of the experimental evidence. https://www.nber.org/papers/w27476
You must be logged in to post a comment.